News
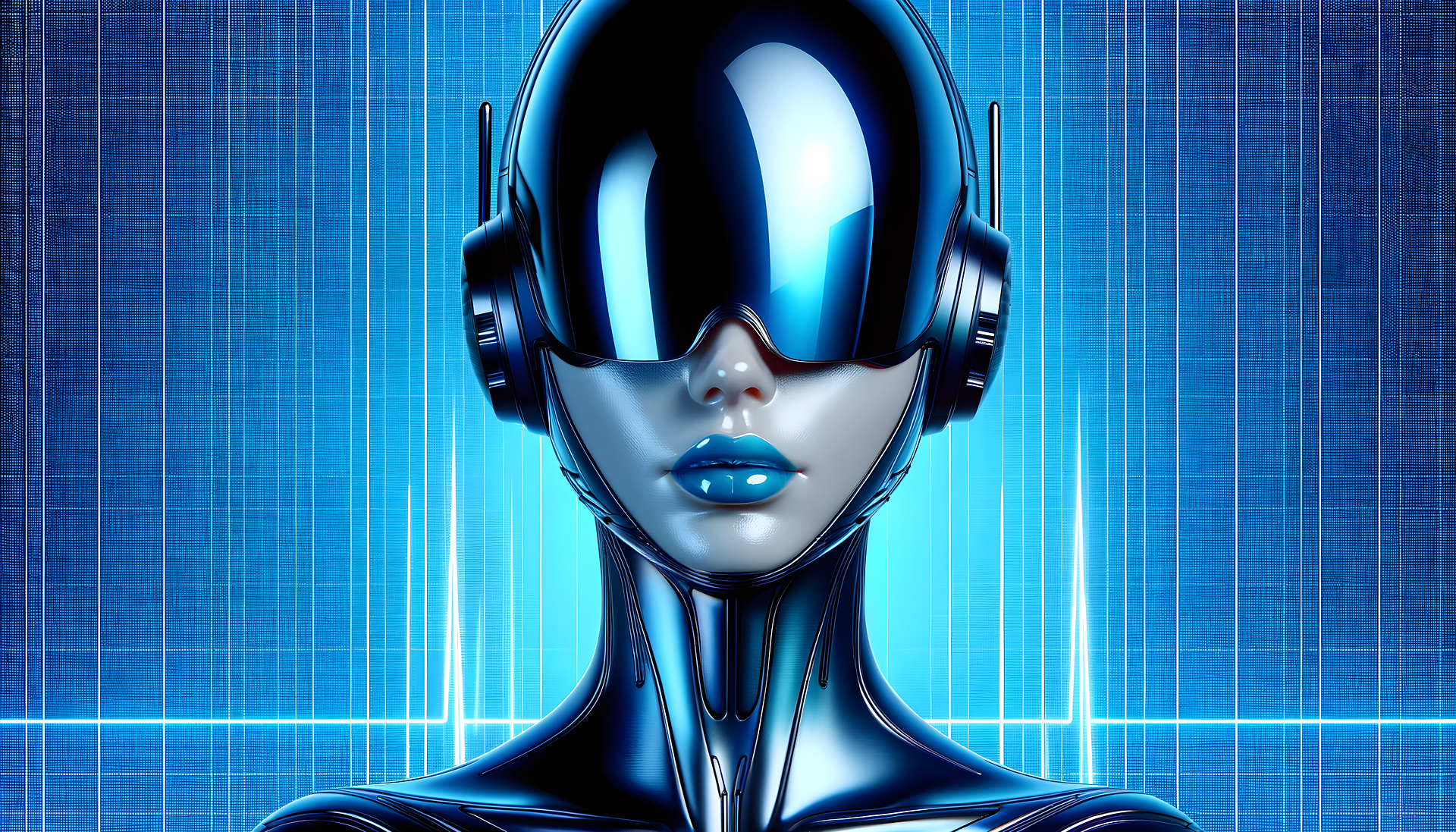
The Rise of Agentic AI
The pace of AI innovation in 2025 is unlike anything we’ve seen before. Among the most transformative developments reshaping the data and AI landscape is the emergence of Agentic AI—a new paradigm that blends large language models with goal-oriented autonomy. For data professionals, data scientists, and AI engineers, understanding this shift is crucial to harnessing the next wave of intelligent automation.
What is Agentic AI?
Agentic AI refers to AI systems designed to operate as autonomous “agents,” capable of understanding goals, making decisions, executing complex multi-step tasks, and learning from interactions—all with minimal human input. These agents go beyond static prompts or model outputs; they can navigate APIs, write code, retrieve data, and iterate based on feedback to complete tasks that traditionally required human oversight.
At its core, Agentic AI combines the power of LLMs (Large Language Models) like GPT-4 and Claude with tool use, planning frameworks, and memory systems. Think of it as moving from a smart assistant to an intelligent collaborator that can manage entire workflows.
Why It Matters to Data Professionals
The rise of Agentic AI is a potential game-changer across the entire data value chain:
-
Data Engineering: Agentic agents can autonomously manage data pipeline health, rerun failed jobs, and even refactor code. By integrating with systems like Airflow, dbt, or Snowflake, they provide continuous optimization and real-time remediation.
-
Data Science & ML: Imagine agents that can run exploratory data analysis, build baseline models, tune hyperparameters, and generate reports—freeing up data scientists to focus on strategic modeling and interpretation.
-
Data Governance & Quality: Agentic systems can monitor for schema drift, detect anomalies, and generate alerts or fixes in real-time. With the right configuration, they act as proactive stewards of data quality and compliance.
-
Business Intelligence: Agentic AI can answer natural language questions over dashboards, generate insights on demand, and automate repetitive reporting tasks—bridging the gap between business users and data assets.
Real-World Use Cases Emerging in 2025
-
AutoML Agents: Automatically orchestrating model selection, training, evaluation, and deployment pipelines with just a high-level goal provided by a user.
-
ETL Maintenance Bots: Monitoring data pipelines for bottlenecks or anomalies, then auto-suggesting or applying improvements without engineering intervention.
-
Autonomous Data Documentation: Generating and updating metadata, lineage, and wiki entries as new datasets and transformations are introduced.
-
AI Agents for Compliance: Continuously auditing data access, detecting policy violations, and suggesting or implementing access changes in accordance with regulatory frameworks like GDPR or HIPAA.
Challenges and Considerations
With this new capability comes new responsibility. Agentic AI systems must be auditable, safe, and aligned with organizational goals. For data leaders, it’s essential to invest in robust monitoring, clear human-in-the-loop processes, and AI governance frameworks. While these agents offer enormous efficiency gains, they also raise questions around accountability, data integrity, and unintended consequences.
Final Thoughts
Agentic AI is not science fiction—it’s already here, reshaping how we think about automation, intelligence, and the role of data teams. As the ecosystem matures, professionals who understand how to leverage, manage, and ethically deploy these agents will be at the forefront of innovation.
For data professionals looking to stay competitive, now is the time to explore the tools and platforms supporting Agentic AI. Whether it’s integrating agents into your workflows or upskilling in prompt engineering and agent orchestration, the opportunity to lead in this new AI era is wide open.